Dedicated to reform of Arkansas family law. In addition to detailing legal arguments, I include subjects that enrich a child's life.
Friday, November 21, 2014
Monday, November 17, 2014
Friday, November 14, 2014
Sunday, November 9, 2014
Northrop Grumman sets record with terahertz IC amplifier
31 October 2014
Northrop Grumman sets record with terahertz IC amplifier
Northrop Grumman Corp of Redondo Beach, CA, USA has developed the world's fastest integrated circuit amplifier, which has been recognized by Guinness World Records. The amplifier uses 10 transistor stages to reach an operating frequency of 1 trillion cycles per second (1 terahertz, or 1012Hz) — surpassing the firm’s own record of 850 billion cycles per second (850GHz) set in 2012.
Researchers have long sought to exploit the terahertz high-frequency band lying between microwaves and infrared light waves in the electromagnetic spectrum, but have been unable to detect, process and radiate the necessary high-frequency signals without resorting to frequency translation or multiplication (hence the term ‘terahertz gap’).
Northrop Grumman’s terahertz monolithic integrated circuit (TMIC) effectively bridges the gap by using a super-scaled 25nm gate-length indium phosphide (InP) high-electron-mobility transistor (HEMT) that measures at a gain of 10dB at 1THz and 9dB at 1.03THz. In comparison, existing smartphones operate at 1-2GHz and wireless networks at 5.7GHz.
The circuit is the culmination of the firm’s three-phase contract with the US Defense Advanced Research Projects Agency (DARPA) to demonstrate transistor-based electronics operating at 670GHz, 850GHz and 1THz. All three milestones were completed by Northrop Grumman within five years.
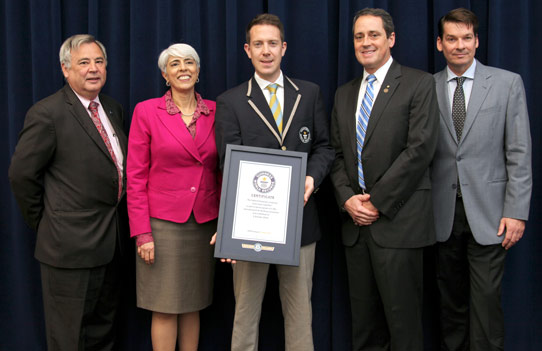
Picture: Philip Robertson (center), representing Guinness World Records, presents the certificate for the fastest integrated circuit amplifier to (from left): Dr Dale Burton, sector VP & chief technology officer Northrop Grumman Aerospace Systems; DARPA director Dr Arati Prabhakar; DARPA’s Terahertz Electronics program manager Dr Dev Palmer; and Northrop Grumman’s Terahertz Electronics program manager Dr William Deal.
“This breakthrough by the Northrop Grumman team could lead to revolutionary technologies such as high-resolution security imaging systems, improved collision-avoidance radar, communications networks with many times the capacity of current systems, and spectrometers that could detect potentially dangerous chemicals and explosives with much greater sensitivity,” comments DARPA’s Terahertz Electronics program manager Dr Dev Palmer.
Additional applications of the THz circuit could include atmospheric sensing, radio astronomy and medical imaging. It is also expected to improve system range, and to reduce the size, weight and power consumption of existing systems.
“A decade ago, there was no consensus in the scientific community whether an integrated circuit operating at 1 terahertz was technologically possible,” says Northrop Grumman’s Terahertz Electronics program manager Dr William Deal. “An interdisciplinary team of scientists and engineers worked together in scaling all facets of our MMIC technology to enable this result,” he adds. “Now, as a result of DARPA’s investment in high-speed transistor processes, it will become routine to fabricate wafers containing thousands of terahertz integrated circuits.”
DARPA has made strategic investments in terahertz electronics through its High Frequency Integrated Vacuum Electronics (HiFIVE), Sub-millimeter Wave Imaging Focal Plane Technology (SWIFT) and Technology for Frequency Agile Digitally Synthesized Transmitters (TFAST) programs. Each program built on the successes of the previous program, providing the foundational research necessary for frequencies to reach the terahertz threshold. Northrop Grumman has partnered with DARPA on each program.
See related items:
Tags: Northrop Grumman InP HEMT
Visit: www.northropgrumman.com/mps
Visit: www.darpa.mil
Saturday, November 8, 2014
fMRI Data Reveals the Number of Parallel Processes Running in the Brain
Emerging Technology From the arXiv
November 5, 2014
fMRI Data Reveals the Number of Parallel Processes Running in the Brain
The human brain carries out many tasks at the same time, but how many? Now fMRI data has revealed just how parallel gray matter is.

The human brain is often described as a massively parallel computing machine. That raises an interesting question: just how parallel is it?
Today, we get an answer thanks to the work of Harris Georgiou at the National Kapodistrian University of Athens in Greece, who has counted the number of “CPU cores” at work in the brain as it performs simple tasks in a functional magnetic resonance imaging (fMRI) machine. The answer could help lead to computers that better match the performance of the human brain.
The brain itself consists of around 100 billion neurons that each make up to 10,000 connections with their neighbors. All of this is packed into a structure the size of a party cake and operates at a peak power of only 20 watts, a level of performance that computer scientists observe with unconcealed envy.
fMRI machines reveal this activity by measuring changes in the levels of oxygen in the blood passing through the brain. The thinking is that more active areas use more oxygen so oxygen depletion is a sign of brain activity.
Typically, fMRI machines divide the brain into three-dimensional pixels called voxels, each about five cubic millimeters in size. The complete activity of the brain at any instant can be recorded using a three-dimensional grid of 60 x 60 x 30 voxels. These measurements are repeated every second or so, usually for tasks lasting two or three minutes. The result is a dataset of around 30 million data points.
Georgiou’s work is in determining the number of independent processes at work within this vast data set. “This is not much different than trying to recover the (minimum) number of actual ‘cpu cores’ required to ‘run’ all the active cognitive tasks that are registered in the entire 3-D brain volume,” he says.
This is a difficult task given the size of the dataset. To test his signal processing technique, Georgiou began by creating a synthetic fMRI dataset made up of eight different signals with statistical characteristics similar to those at work in the brain. He then used a standard signal processing technique, called independent component analysis, to work out how many different signals were present, finding that there are indeed eight, as expected.
Next, he applied the same independent component analysis technique to real fMRI data gathered from human subjects performing two simple tasks. The first was a simple visuo-motor task in which a subject watches a screen and then has to perform a simple task depending on what appears.
In this case, the screen displays either a red or green box on the left or right side. If the box is red, the subject must indicate this with their right index finger, and if the box is green, the subject indicates this with their left index finger. This is easier when the red box appears on the right and the green box appears on the left but is more difficult when the positions are swapped. The data consisted of almost 100 trials carried out on nine healthy adults.
The second task was easier. Subjects were shown a series of images that fall into categories such as faces, houses, chairs, and so on. The task was to spot when the same object appears twice, albeit from a different angle or under different lighting conditions. This is a classic visual recognition task.
The results make for interesting reading. Although the analysis is complex, the outcome is simple to state. Georgiou says that independent component analysis reveals that about 50 independent processes are at work in human brains performing the complex visuo-motor tasks of indicating the presence of green and red boxes. However, the brain uses fewer processes when carrying out simple tasks, like visual recognition.
That’s a fascinating result that has important implications for the way computer scientists should design chips intended to mimic human performance. It implies that parallelism in the brain does not occur on the level of individual neurons but on a much higher structural and functional level, and that there are about 50 of these.
Georgiou points out that a typical voxel corresponds to roughly three million neurons, each with several thousand connections with its neighbors. However, the current state-of-the-art neuromorphic chips contain a million artificial neurons each with only 256 connections. What is clear from this work is that the parallelism that Georgiou has measured occurs on a much larger scale than this.
“This means that, in theory, an artificial equivalent of a brain-like cognitive structure may not require a massively parallel architecture at the level of single neurons, but rather a properly designed set of limited processes that run in parallel on a much lower scale,” he concludes.
Anybody thinking of designing brain-like chips might find this a useful tip.
Ref: arxiv.org/abs/1410.7100 Estimating The Intrinsic Dimension In fMRI Space Via Dataset Fractal Analysis
Subscribe to:
Posts (Atom)